History of OCR
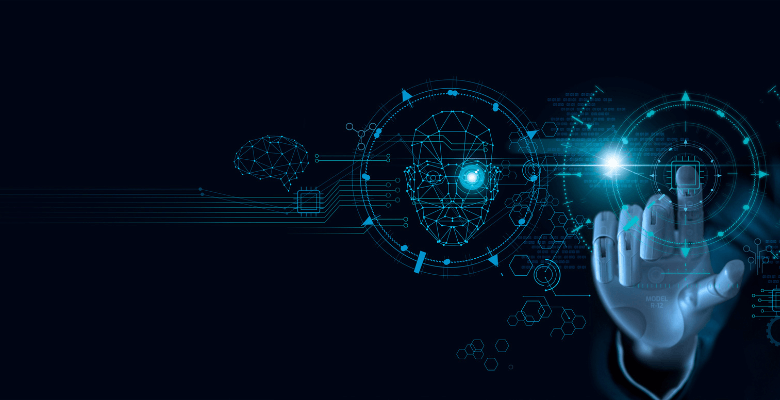
OCR technology in its early stages was primarily focused on recognizing specific fonts or characters. However, the technology continued to evolve over the decades, incorporating advancements in electronics, computing power, and machine learning to become the sophisticated text recognition systems we have today.
OCR, or Optical Character Recognition, is a technology that converts printed or handwritten text into machine-readable digital text. It enables automation in tasks like data entry, document processing, and text extraction from images, improving efficiency in various industries.
The history of OCR allows us to appreciate the significant technological progress it has made. For instance, early OCR struggled with recognizing handwritten text and complex fonts. Knowing this history informs us about the challenges that remain today, like recognizing handwriting or text within complex layouts.
Likewise, from rudimentary character recognition to advanced deep learning models, OCR has come a long way. This context helps us appreciate the complexity of modern OCR systems.
Milestones in OCR Development
Early Mechanical OCR Devices (Early 20th Century):
In the early 1900s, inventors like Emanuel Goldberg pioneered the development of mechanical OCR devices. Goldberg’s “Reading Machine” was a notable example.
These machines used arrays of light-sensitive cells and complex mechanical components to recognize printed characters on paper.
While limited in scope and accuracy, they laid the foundation for the concept of mechanically recognizing printed text, showcasing the potential of automation in text processing.
Emergence of Electronic OCR (1940s-1950s):
After World War II, the development of electronic components and computers gave rise to more advanced OCR systems. Dr. Edmund F. Callaghan’s “Statistical Machine,” created in the late 1930s, represented an early commercial OCR device. It was designed for reading numbers and letters on documents.
These electronic systems marked a shift away from purely mechanical solutions, offering increased speed and efficiency in character recognition.
Advancements in Pattern Recognition (1960s-1970s):
The 1960s and 1970s saw significant progress in pattern recognition algorithms, which are essential for OCR accuracy. Researchers focused on improving the recognition of characters by developing more robust algorithms capable of handling variations in fonts, sizes, and styles.
Commercial OCR systems began to emerge, offering greater accuracy and reliability for character recognition tasks.
Digital OCR and Computer Processing (1980s-1990s)
The advent of personal computers in the 1980s marked a pivotal moment for OCR technology. Optical scanners became more accessible and affordable, allowing users to scan printed documents and use OCR software to extract text.
OCR transitioned from being predominantly hardware-based to software-driven, making it easier for businesses and individuals to utilize text recognition capabilities.
Integration of Machine Learning (2000s-2010s):
In the 2000s, machine learning techniques, particularly neural networks, began to play a significant role in OCR development. These techniques improved OCR’s ability to recognize text by learning from vast datasets and adapting to different fonts, languages, and styles.
OCR systems became more versatile and accurate, making them suitable for a wider range of applications.
Mobile OCR and Real-Time Processing (2010s):
The 2010s brought the rise of mobile OCR apps, allowing users to extract text from images using smartphones and tablets. Real-time OCR processing became feasible, enabling instant text recognition and translation using mobile devices.
This innovation revolutionized tasks like text translation, data capture, and accessibility for people with visual impairments.
Deep Learning and AI (2010s-Present):
Deep learning approaches, particularly convolutional neural networks (CNNs), have been pivotal in OCR’s recent advancements. These AI-driven OCR systems can handle complex documents with varied layouts, fonts, and languages, achieving remarkable accuracy.
OCR technology is no longer limited to recognizing specific fonts. AI-driven OCR systems can identify and interpret text in a wide range of fonts and styles, including handwritten text and decorative fonts.
OCR has become integral to automation in industries such as finance, healthcare, and logistics, where text data extraction is critical. Their ability to adapt, learn, and recognize text accurately in a wide range of scenarios has made them indispensable tools in industries where text data extraction and processing are critical. These advancements have not only improved efficiency but have also expanded accessibility and usability for a broader audience
How OCR Technology is Used Today: Integrations
Due to their accuracy and adaptability, OCR systems have multiple applications across industries. They are used in document management, data extraction, automated data entry, and more, streamlining processes and reducing manual labor.
Here’s how OCR technology is used in a variety of applications:
Finance and Banking
Automated Data Entry: OCR is used to extract data from financial documents like invoices, receipts, and checks, reducing manual data entry errors.
Document Classification: It categorizes and extracts information from loan applications and mortgage documents for faster processing.
Fraud Detection: OCR assists in comparing and verifying text data to detect potential fraud in financial transactions.
Healthcare
Electronic Health Records (EHR) Management: OCR digitizes patient records, making them accessible electronically for healthcare providers.
Prescription Data Extraction: It automates the extraction of prescription information for efficient pharmacy management.
Medical Billing: OCR simplifies the processing of medical billing and insurance claims by extracting relevant data.
Legal
Document Digitization: Legal documents are scanned and digitized, enabling electronic case management and easier document retrieval.
Automated Indexing: OCR helps in indexing and searching legal texts for efficient legal research.
Handwritten Text Conversion: OCR can convert handwritten legal notes into digital and searchable format.
Retail and E-commerce
Inventory Management: OCR is used for inventory management by digitizing product labels and barcodes.
Automated Invoice Processing: It automates the processing of invoices, receipts, and purchase orders for faster payment and inventory tracking.
Competitor Analysis: OCR extracts price and product information from competitor advertisements for market analysis.
Logistics and Supply Chain
Data Capture: OCR captures data from shipping labels and packing slips for accurate tracking.
Barcode Scanning: It facilitates inventory tracking through OCR-enabled barcode scanning.
Shipping Information Extraction: OCR extracts shipping and delivery information for logistics management.
Seeking a Powerful OCR Solution for Your Business?
As we’ve seen, OCR has evolved from its early mechanical experiments to sophisticated AI-driven systems capable of handling complex layouts, fonts, and languages with remarkable accuracy.
For businesses and organizations seeking a powerful OCR solution to streamline data extraction and document processing, we introduce Klearstack.
Klearstack’s OCR solution offers a range of benefits:
- Exceptional 99% accuracy with AI-driven OCR.
- Up to 70% cost savings compared to manual entry.
- Quick and hassle-free setup with no templates required.
- Saves thousands of hours of manual effort and costs.
- Versatile data extraction from various document formats.
Simplify your tasks with KlearStack. Extract data from invoices easily, efficiently, and accurately.